The Book of Why: How a ‘causal revolution’ is shaking up science
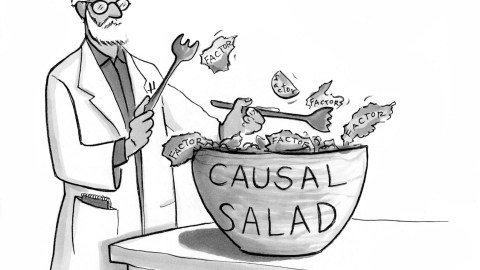
1. The Book of Why brings a “new science” of causes. Judea Pearl’s causology graphically dispels deep-seated statistical confusion (but heterogeneity-hiding abstractions and logic-losing numbers lurk).
2. Pearl updates old correlation-isn’t-causation wisdom with “causal questions can never be answered from data alone.” Sorry, Big Data (and A.I.) fans: “No causes in, no causes out” (Nancy Cartwright).
3. Because many causal processes can produce the same data/stats, it’s evolutionarily fitting that “the bulk of human knowledge is organized around causal, not probabilistic relationships.” Crucially, Pearl grasps that “the grammar of probability [& stats]… is insufficient.”
4. But trad stats isn’t causal “model-free,” it implicitly imposes “causal salad” models—independent factors, jumbled, simple additive effects (widely method-and-tool presumed … often utterlyunrealistic).
5. “Causal revolution” methods enable richer logic than trad-stats syntax permits (for instance, arrowed-line causal structure diagrams enhance non-directional algebra).
6. Paradoxically, precise-seeming numbers can generate logic-fogging forces. The following reminders might counter rote-method-produced logic-losing numbers.
7. Causes of changes in X, need not be causes of X. That’s often obvious in known-causality cases (pills lowering cholesterol aren’t its cause) but routinely obfuscated in analysis-of-variance research. Correlating variation percentages to factor Y often doesn’t “explain” Y’s role (+see “red brake risk”). And stats factor choice can reverse effects (John Ioannidis).
8. Analysis-of-variance training encourages fallacy-of-division miscalculations. Many phenomena are emergently co-caused and resist meaningful decomposition. What % of car speed is “caused’ by engine or fuel? What % of drumming is “caused” by drum or drummer? What % of soup is “caused” by its recipe?
9. Akin to widespread statistical-significance misunderstandings, lax phrasing like “control for” and “held constant” spurs math-plausible but impossible-in-practice manipulations (~“rigor distoris”).
10. Many phenomena aren’t causally monolithic “natural kinds.” They evade classic causal-logic categories like “necessary and sufficient,” by exhibiting “unnecessary and sufficient” cause. They’re multi-etiology/route/recipe mixed bags (see Eiko Fried’s 10,377 paths to Major Depression).
11. Mixed types mean stats-scrambling risks: fruitless apples-to-oranges stats like average humans have 1 testicle + 1 ovary.
12. Pearl fears trad-stats-centric probability-intoxicated thinking hides its staticness, whereas cause-driven approaches illuminate changing scenarios. Causality always beats stats (which encode unnovel cases). Known causal-composition rules (your system’s syntax) make novel (stats-defying) cases solvable.
13. “Causal revolution” tools overcome severe trad-stats limits, but they retain rush-to-the-numbers risks (is everything relevant squeezable into path-coefficients?) and type-mixing abstractions (e.g., Pearl’s diagram lines treat them equivalently but causes work differently in physics versus social systems).
14. “Cause” is a suitcase concept, requiring a richer causal-role vocabulary. Recall Aristotle’s cause kinds—material, formal, proximate, ultimate. Their qualitative distinctness ensures quantitative incomparability. They resist squashing into a single number (ditto needed Aristotle-extending roles).
15. Causal distance always counts. Intermediate-step unknowns mean iffier logic/numbers (e.g., genes typically exert many-causal-steps-removed highly co-causal effects).
16. Always ask: Is a single causal structure warranted? Or casual stability? Or close-enough causal closure? Are system components (roughly) mono-responsive?
17. Skilled practitioners respect their tools’ limits. A thinking-toolkit of context-matched rule-of-thumb maxims might counter rote-cranked-out methods and heterogeneity-hiding logic-losing numbers.