Effective machine learning needs leadership — not AI hype
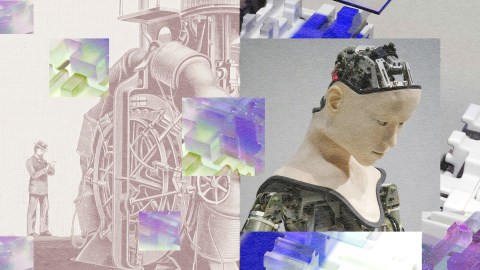
- The world largely knows of machine learning (ML) as AI — but the term AI is also how the world largely misunderstands ML.
- Glamorizing core ML technology takes the focus off its concrete value.
- A greater problem than overselling ML is the dramatic downplaying of deployment complexity.
The ML (Machine Learning) industry has bitten forbidden fruit: It has chosen to promote itself as AI, an ill-defined umbrella term that includes ML within its malleable scope. This tends to mislead, especially when discussing a more typical, practical ML initiative designed to improve business operations and not, for example, meant to generate humanlike writing or to achieve human-level “intelligence.”
While the world largely knows of ML as AI, the term AI is also how the world largely misunderstands ML. Because AI alludes to “intelligence,” which is stubbornly nebulous when describing a technology, the term tends to overstate and fetishize rather than pitching the technology’s concrete value. AI is sometimes used to specifically refer to ML or another kind of technology like chatbots or rule-based systems—but in many other uses, the term hints at exaggerated capabilities.
Vendors, consultants, and, chances are, some of your colleagues employ the AI brand rather than clearly advertising, without obfuscation, what an ML project actually offers. After all, plenty of folks with a budget have ears that perk up when they hear how advanced and “intelligent” a technology is, even without seeing precisely how it will improve business operations. So that route could serve to pad your wallet, at least in the short term.
But it can’t last. The ML industry had better tone this down or we’re all going to pay dearly. Glamorizing the core technology takes the focus off its concrete value, the specific way its deployment can improve operations. When that deployment isn’t central to the plan, the plan is unlikely to come to fruition. Instead, the organization must consider the value proposition for a candidate project and buy into the project for that tangible value. Then, a very particular change management process must commence from the project’s onset. Otherwise, you’re prone to develop a model that never gets launched—which is the most common way ML projects fail.
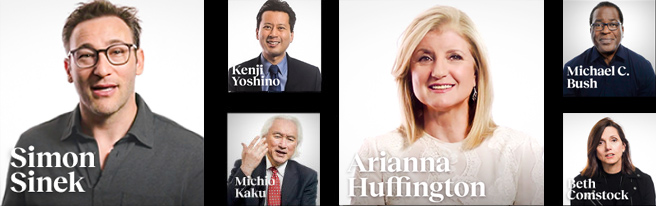
Capitalizing on this technology is critical—but it’s notoriously difficult to launch. Many ML projects never progress beyond the modeling: the number-crunching phase. Industry surveys repeatedly show that most new ML initiatives don’t make it to deployment, where the value would be realized.
Hype contributes to this problem. ML is mythologized, misconstrued as “intelligent” when it is not. It’s also mismeasured as “highly accurate,” even when that notion is irrelevant and misleading. For now, these adulations largely drown out the words of consternation, but those words are bound to increase in volume.
Take self-driving cars. In the most publicly visible cautionary tale about ML hype, overzealous promises have led to slamming on the brakes and slowing progress. As The Guardian put it, “The driverless car revolution has stalled.” This is a shame, as the concept promises greatness. Someday, it will prove to be a revolutionary application of ML that greatly reduces traffic fatalities. This will require a lengthy “transformation that is going to happen over 30 years and possibly longer,” according Chris Urmson, formerly the CTO of Google’s self-driving team and now the CEO of Aurora, which bought out Uber’s self-driving unit. But in the mid-2010s, the investment and fanatical hype, including grandiose tweets by Tesla CEO Elon Musk, reached a premature fever pitch. The advent of truly impressive driver assistance capabilities were branded as “Full Self-Driving” and advertised as being on the brink of widespread, completely autonomous driving—that is, self-driving that allows you to nap in the back seat.
Expectations grew, followed by . . . a conspicuous absence of self-driving cars. Disenchantment took hold and by the early 2020s investments had dried up considerably. Self-driving is doomed to be this decade’s jetpack.
What went wrong? Underplanning is an understatement. It wasn’t so much a matter of overselling ML itself, that is, of exaggerating how well predictive models can, for example, identify pedestrians and stop signs. Instead, the greater problem was the dramatic downplaying of deployment complexity. Only a comprehensive, deliberate plan could possibly manage the inevitable string of impediments that arise while slowly releasing such vehicles into the world. After all, we’re talking about ML models autonomously navigating large, heavy objects through the midst of our crowded cities! One tech journalist poignantly dubbed them “self-driving bullets.” When it comes to operationalizing ML, autonomous driving is literally where the rubber hits the road. More than any other ML initiative, it demands a shrewd, incremental deployment plan that doesn’t promise unrealistic timelines.
The ML industry has nailed the development of potentially valuable models, but not their deployment.
The ML industry has nailed the development of potentially valuable models, but not their deployment. A report prepared by the AI Journal based on surveys by Sapio Research showed that the top pain point for data teams is “Delivering business impact now through AI.” Ninety-six percent of those surveyed checked that box. That challenge beat out a long list of broader data issues outside the scope of AI per se, including data security, regulatory compliance, and various technical and infrastructure challenges. But when presented with a model, business leaders refuse to deploy. They just say no. The disappointed data scientist is left wondering, “You can’t . . . or you won’t?” It’s a mixture of both, according to a question asked by my survey with KDnuggets (see responses to the question, “What is the main impediment to model deployment?”). Technical hurdles mean that they can’t. A lack of approval—including when decision makers don’t consider model performance strong enough or when there are privacy or legal issues—means that they won’t.
Another survey also told this “some can’t and some won’t” story. After ML consultancy Rexer Analytics’ survey of data scientists asked why models intended for deployment don’t get there, founder Karl Rexer told me that respondents wrote in two main reasons: “The organization lacks the proper infrastructure needed for deployment” and “People in the organization don’t understand the value of ML.”
Unsurprisingly, the latter group of data scientists—the “won’ts” rather than the “can’ts”—sound the most frustrated, Karl says.
Whether they can’t or they won’t, the lack of a well-established business practice is almost always to blame. Technical challenges abound for deployment, but they don’t stand in the way so long as project leaders anticipate and plan for them. With a plan that provides the time and resources needed to handle model implementation—sometimes, major construction—deployment will proceed. Ultimately, it’s not so much that they can’t but that they won’t.