Researchers use AI to predict hit songs from listeners’ heart rates
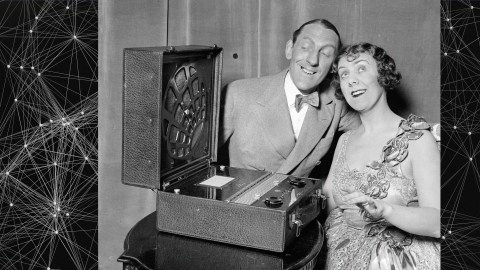
- Predicting which new songs will become hits is notoriously difficult.
- New research shows that machine learning algorithms can accurately predict hit songs from neural activity inferred from heart rates alone.
- The technique, known as “neuroforecasting,” might also be used to make predictions about stock market behavior.
Every week, nearly 170,000 new songs are released worldwide, but as anyone in the music industry will tell you, predicting which of those will be successful is practically impossible. The answer to this so-called “Hit Song Science” problem may lie in the brain. According to new research published in the journal Frontiers in Artificial Intelligence, machine learning algorithms can analyze neural responses to songs to predict accurately which ones will be hits.
Song science
Sean Merritt of Claremont Graduate University and his colleagues recruited 33 volunteers and recorded their heart rate while they listened to 24 songs chosen independently by the staff at a streaming service. The data was then fed into a model that can predict brain activity from changes in heart rate.
All the songs had been released within the previous six months and spanned a variety of genres, including electronic dance music, hip hop, and rock. After hearing each song, the participants completed a simple survey to rank how much they liked it (on a scale from one to ten) and whether they would recommend it to their friends. The researchers also used metrics from the streaming service to categorize the songs as “hits” or “flops.” Thirteen of them had been streamed more than 700,000 times and were deemed “hits,” while the rest had been streamed far fewer times and were considered “flops.”
Comparing the self-reports to the streaming metrics, Merritt and his colleagues found that, for songs the participants were familiar with, their reports of how much they liked it were closely related to the number of times it had been streamed. But when they analyzed unfamiliar songs, this relationship disappeared, and the participants’ self-reports did not accurately predict which songs were hits.
Using the participants’ neural responses (which were inferred from their heart rate alone), the researchers looked for signs of attention and emotion and used those two measures to calculate an “immersion” value for each song, which can be loosely defined as a measure of how engaging the participants found it. Using statistical methods to analyze the responses, they found that they could predict hit songs — that is, those with the highest immersion values — with an accuracy of 69%. When they trained a machine learning algorithm using a larger synthetic data set, the accuracy of the predictions increased to 97%.
Neuroforecasting
The study is the latest in an emerging field known as “neuroforecasting,” which aims to explore if brain activity collected from small groups of people can be used to predict behavior or choices in the wider population.
Several years ago, researchers at Stanford University demonstrated that brain activity can reveal “hidden” information that can predict which YouTube videos will go viral. More recently, a study carried out at the Rotterdam School of Management showed that the neural responses of professional investors seem to predict the future market performance of stocks.
There have been previous attempts to predict the popularity of new music from brain activity, but none has approached the level of accuracy reached in this present study. Yet, the researchers emphasize that their sample size was small, making it unclear if the findings will generalize to larger song catalogs. Nevertheless, if the findings are replicated, neurotechnology-based artificial intelligence could soon be used to curate playlists and would likely replace and improve existing recommendation algorithms.