From drugs to chemical weapons with the flip of an AI switch
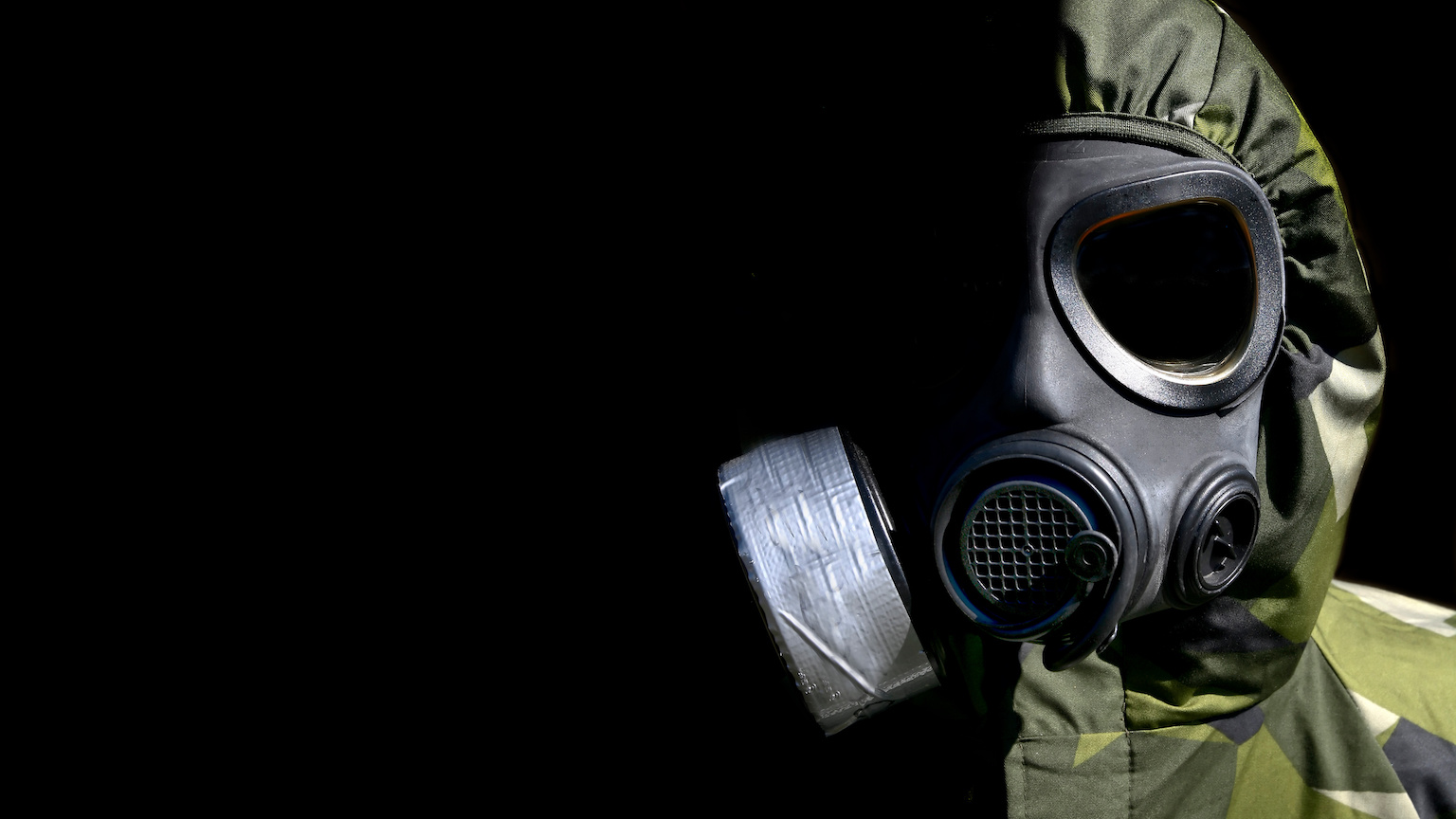
- Like its name implies, dual-use technologies can serve two purposes: one good, the other evil.
- AI chemical discovery is one such technology. It can be used to discover new medicines — or chemical weapons.
- One research group found that their AI, in under six hours, designed VX nerve agent and 40,000 other similar molecules.
In 2017, the half-brother of North Korean leader Kim Jong-un was poisoned with VX at the Kuala Lumpur airport. VX is a nerve agent that induces uncontrollable clenching of the muscles such that the victim cannot breathe, leading to death within a few minutes or hours.
North Korea isn’t the only country known to make or use deadly chemicals like VX. But if you think designing chemical warfare agents requires the infrastructure and research capabilities of a nation-state, you are in for a shock.
The Spiez Laboratory — a Swiss institute that monitors the threat of nuclear, biological, and chemical weapons — organizes a biennial conference that explores new chemical and biological technologies that may pose a security risk in the hands of malicious actors.
Last year, one of the participants at the conference was Collaboration Pharmaceuticals, a U.S.-based pharma company that was asked to discuss how their tech for AI-based drug discovery could be misused.
They showed that, with some tweaks, their AI models could be repurposed to do the opposite of what they were created for — instead of discovering new treatments, they could create thousands of brand new chemical weapons.
Designing chemical warfare agents does not require the infrastructure and research capabilities of a nation-state
Traversing the chemical space
The number of potential ways different elements can be linked to each other is endless.
The chemical space — a theoretical representation of the entire universe of all possible chemicals — comprises 10180 compounds, far greater than the number of atoms in the universe (1080).
Like the physical universe, it is sparse — most theoretical configurations are not realistically possible. But artificial intelligence algorithms are now opening the window to what’s achievable in chemical space.
However, even with AI, traversing the whole chemical space is an inefficient approach to discovering new chemicals. Most potential chemicals would be highly unstable, hard to synthesize, lack the desired properties, or have undesirable properties.
This is why computational chemists add constraints to their AI algorithms to find what they are looking for faster.
The number of ways elements can be linked together is endless.
These constraints are defined by the nature of their application. For instance, a drug design team will optimize its algorithms to look for chemicals with the potential to cause a particular reaction, such as binding to a receptor or inhibiting a bimolecular reaction, while not being toxic to the body.
Collaboration Pharmaceuticals, for one, uses machine learning models to avoid molecules that could cause different kinds of toxic reactions.
Designing chemical weapons on your terminal
In the work the company presented at the Spiez conference, now published in Nature Machine Intelligence, they inverted the logic of their machine learning models.
Instead of screening out toxic chemicals, they tweaked the models to actively select for them. In less than 6 hours of runtime on their servers, their reconfigured AI models designed not just VX, but 40,000 similar molecules, both with publicly available structures and completely novel ones. Many of these were far more toxic than publicly known chemical weapons.
The researchers involved in the study stated that they had “transformed our innocuous generative model from a helpful tool of medicine to a generator of likely deadly molecules.”
The most alarming takeaway from this little experiment was how easy it was to execute. The barrier to entry for designing chemicals with desired properties is far lower than it has ever been. While knowledge of chemistry still helps, it is only supplementary (at least in the design part of the process) when computers do the heavy lifting.
Collaboration Pharmaceuticals is just one of hundreds or thousands of companies with the capability to design chemicals like this. With open-source models and publicly accessible toxicity databases, it is easy for anyone with the intent and the computing resources to replicate this experiment.
Many of the compounds the AI designed were far more toxic than publicly known chemical weapons.
In the universe of chemical compounds, there are far more potential toxic molecules than are already known to us. With some tweaks in the toxicity prediction models, like the way it was done in this study, it would be possible to access these.
Going beyond design, cloud manufacturing makes the synthesis of designer chemicals accessible to anyone with a computer and an Internet connection.
The dual-use potential of generative models
Commentators often warn against the risks of artificial intelligence. However, most of these discussions on the dual-use of AI are centered around data safety, privacy, discriminatory algorithms, and the like. The potential of misusing generative models to create chemical or biological weapons necessitates that we talk about the national and international security risks that AI poses.
The authors highlight the irony in how GPT-3, a generative model that can write viral blog posts, comes with a filter for insensitive language, but toxicity or chemical target modeling algorithms are available without any guardrails. Pharma companies aren’t the only ones developing AI models for chemical discovery and design. Materials, agrochemicals, and even food companies are using generative chemistry, all without much thought about the potential for these AI models to be misused.
If a bad-faith actor were to use these models to design highly potent chemical weapons or, worse, use them, it could be unlike any chemical attack we have seen before. Such an event could harm the reputation of AI as well, causing further damage by draining funding out of the field and limiting its ability to do good — designing new, safer, better drugs and materials. Companies and researchers working on AI-based generative models need to proactively come up with checks and countermeasures, for the better of both humanity and themselves.
This article was originally published by our sister site, Freethink.