A.I. can predict your future tweets by looking at your friends’ accounts
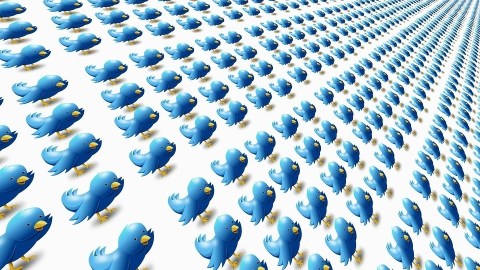
Pixabay
- The study examined the past tweets of hundreds of Twitter users, each within a particular social circle, in order to see how accurate of predictions it’s possible to make with machine-learning techniques.
- The results showed that machine-learning techniques can predict the future Twitter activity of a given user by looking only at about 9 of their friends, and that these predictions are about as accurate as predictions made with the benefit of looking at the user’s past writings.
- The findings have troubling implications for online privacy, namely that, in theory, not even leaving Facebook or Twitter would prevent someone from generating an accurate profile of you.
It’s probably not much of a surprise to know it’s possible to take all of your past writings from Twitter, have a computer examine them, and then have it make a pretty accurate prediction about the types of content you’re likely to write in the future. What’s more surprising, however, is what a new study shows: It’s possible for an A.I. to accurately predict someone’s Twitter activity by looking only at the online behavior of a handful of that person’s friends.
The results highlight a troubling implication: Not even deleting your Twitter or Facebook account would theoretically prevent someone from developing a profile on you — one that, as the study suggests, could be quite accurate:
“The ability of a machine learning method to accurately profile individuals from their online traces is reflected in the predictability of their written text.”
The study, published in the journal Nature Human Behavior, was designed to find out just how accurate of predictions it’s theoretically possible for machine-learning methods to make about a person’s future social media activity by using data on people within their social network.
“We used some very interesting mathematics from information theory to say: If you had the perfect machine learning method, how well could you do?” lead author James Bagrow, a data scientist at the University of Vermont, told Science Magazine.
Here’s a summary of how a machine-learning technique would make such predictions, as the study authors wrote:
The ability of a machine learning method to accurately profile individuals from their online traces is reflected in the predictability of their written text. Indeed, with a language model trained to predict the words a user will post online, in principle, one can construct a profile of the user by evaluating the likelihoods of various words to be posted, such as terms related to politics. Thus, quantifying the predictive information contained within a user’s text allows us to understand the potential accuracy such methods can potentially achieve given a user’s data.
For the study, the researchers looked at more than 30 million posts from about 13,900 Twitter users, each of whom had 50 to 500 followers. From these data sets they designated 927 so-called ego networks, at the center of which was a Twitter user and about 9 of their most frequently mentioned Twitter contacts. The researchers then looked at all of the users’ past writings, categorizing them based on the contents and timings of the tweets, finding that users typically only used between 45 and 256 different words to compose tweets, which is “far smaller than the typical user’s ~5,000-word vocabulary,” the authors wrote. This limited range makes it relatively easy to predict words users are likely to write.
After conducting various tests, the results showed that machine-learning techniques could achieve 95% potential predictive accuracy for a given person using their social ties alone, without examining that individual’s personal Twitter data. Although the study didn’t actually predict specific tweets, the study illustrates the striking amount of predictive information about us that lies within our social groups.
One interesting note about the findings: Generally, when researchers were gauging the potential predictive accuracy for a given user, the accuracy increased as they added more friends of the user to the mathematical mix. But at a certain point, adding new friends stopped increasing accuracy. Interestingly, the authors wrote that this limit seems compatible with Dunbar’s number, which describes how human beings can generally only maintain about 150 social ties. Or, as Dunbar explained, it’s “the number of people you would not feel embarrassed about joining uninvited for a drink if you happened to bump into them in a bar.”
In online privacy, your friends have control over your data
In an age when many are already worried about the lack of control they wield over their personal data, the study implies that even total control over our own data might be irrelevant if an organization can build an accurate profile of you by using your friends’ data alone. In other words, even leaving Facebook or Twitter theoretically wouldn’t prevent someone gathering reliable information about your political leanings, consumer habits, religious beliefs, etc.
“There’s no place to hide in a social network,” Lewis Mitchell, a co-author on the new study, told The University of Vermont’s UVM Today.
Another implication: When you make your data accessible, you’re also potentially exposing the data of those to whom you’re connected, as Bagrow told Science Magazine:
“When they give up their own data, they’re also giving up data on their friends,” Bagrow said. “What we think is an individual choice in a social network is not really.”
Of course, some people’s online activity is harder to predict than others, and Twitter may prove to be a unique platform in terms of the ability for machine-learning techniques to predict behavior. Still, as long as it’s possible to make such predictions, it’s not hard to see how platforms like Twitter could, either unwittingly or negligently, cause damage by using this kind of information to expand its product, as the authors wrote:
“Language models derived in this way can have important consequences: combining predictions from a language model with an algorithm for recommending new social ties, for example, has the potential to create or exacerbate filter bubbles.”