An AI can read words in brain signals
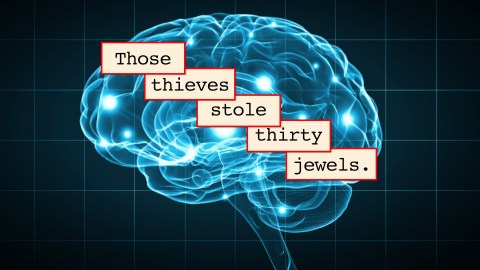
Image source: ESB Professional/Shutterstock/Big Think
It’s just a start, but it’s pretty exciting: a system that translates brain activity into text. For those unable to physically speak, such as people with locked-in syndrome for example, this would be a life-changer.
Right now, it’s a bit like seeing through a heavy fog, but researchers at the Chang Lab at the University of California at San Francisco have trained a machine-learning algorithm to extract meaning from neuronal data.
Joseph Makin, co-author of this research tells The Guardian, “We are not there yet, but we think this could be the basis of a speech prosthesis.”
The research is published in the journal Nature Neuroscience.
Image source: Teeradej/Shutterstock
Eavesdropping
To train their AI, Makin and co-author Edward F. Chang “listened in” on the neural activity of four participants. As epileptics, each participant had had brain electrodes implanted for the purpose of seizure monitoring.
The participants were supplied 50 sentences they were to read aloud at least three times. As they did, neural data was collected by the researchers. (Audio recordings were also made.)
The study lists a handful of the sentences the participants recited, among them:
- “Those musicians harmonize marvelously.”
- “She wore warm fleecy woolen overalls.”
- “Those thieves stole thirty jewels.”
- “There is chaos in the kitchen.”
The algorithm’s task was to analyze the collected neural data and make predictions as to what was being said when the data was generated. (Data associated with non-verbal sounds captured in the participants’ audio recording was factored out first.)
The researchers’ algorithm learned pretty quickly to predict the words associated with chunks of neural data. The AI predicted the data generated when “A little bird is watching the commotion” was spoken would mean “The little bird is watching watching the commotion,” quite close, while “The ladder was used to rescue the cat and the man” was predicted as, “Which ladder will be used to rescue the cat and the man.”
The accuracy varied form participant to participant. Makin and Chang found that an algorithm based on one participant had a head start on being trained for another, suggesting that training the AI could get easier over time and repeated use.
The Guardian spoke with expert Christian Herff, who found the system impressive for using less than 40 minutes of training data for each participant rather than the far greater amount of time required by other attempts to derive text from neural data. He says, “By doing so they achieve levels of accuracy that haven’t been achieved so far.”
Previous attempts to derive speech from neural activity focused on the phonemes from which spoken words are built, but Makin and Chang focused on the overall words instead. While there are certainly more words than phonemes, and thus this poses a greater challenge, the study says, “the production of any particular phoneme in continuous speech is strongly influenced by the phonemes preceding it, which decreases its distinguishability.” To minimize the difficulty of their word-based approach, the spoken sentences used a total of just 250 words.
Image source: whitehoune/Shutterstock/Big Think
Through the neural fog
Clearly, though, there’s room for improvement. The AI also predicted that “Those musicians harmonize marvelously” was “The spinach was a famous singer.” “She wore warm fleecy woolen overalls” was mis-predicted as “The oasis was a mirage.” “Those thieves stole thirty jewels” was misconstrued as “Which theatre shows mother goose,” while the algorithm predicted the data for “There is chaos in the kitchen” meant “There is is helping him steal a cookie.”
Of course, the vocabulary involved in this research is limited, as are the sentence exemplars. “If you try to go outside the [50 sentences used] the decoding gets much worse,” notes Makin, citing the limitations of his study. Another obvious caveat comes from the fact that the AI was trained from sentences spoken aloud by each participant, an impossibility with locked-in patients.
Still, the research by Makin and Chang is encouraging. Predictions for one of their participants required just a tiny 3% correction. That’s actually better than the 5% error rate found in human transcriptions.